Researchers develop traffic prediction system based on neural networks
- Like
- Digg
- Del
- Tumblr
- VKontakte
- Buffer
- Love This
- Odnoklassniki
- Meneame
- Blogger
- Amazon
- Yahoo Mail
- Gmail
- AOL
- Newsvine
- HackerNews
- Evernote
- MySpace
- Mail.ru
- Viadeo
- Line
- Comments
- Yummly
- SMS
- Viber
- Telegram
- Subscribe
- Skype
- Facebook Messenger
- Kakao
- LiveJournal
- Yammer
- Edgar
- Fintel
- Mix
- Instapaper
- Copy Link
Posted: 23 April 2019 | Intelligent Transport | No comments yet
New deep neural networks traffic prediction system uses artificial intelligence to determine when traffic will occur, allowing for predictions 15 minutes ahead of time
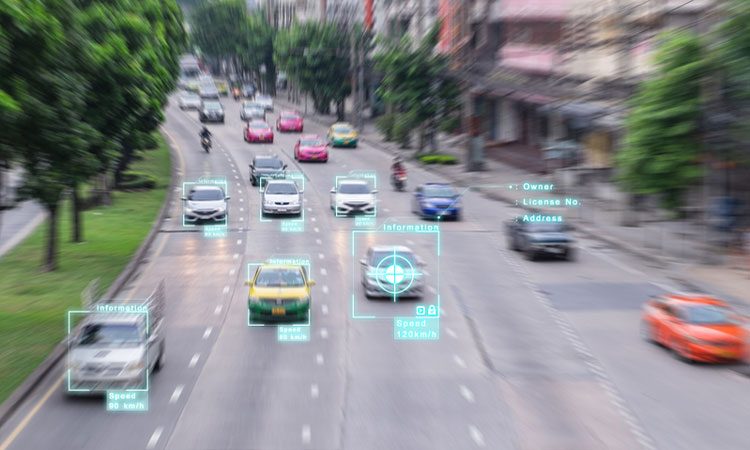
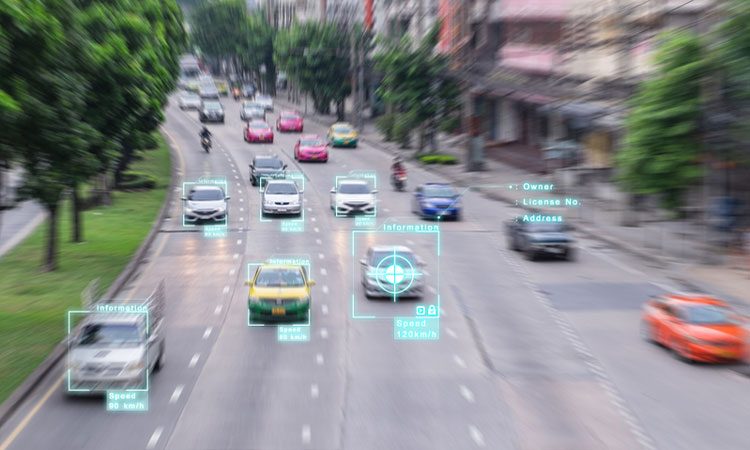
Researchers of the Miguel Hernández University (UMH) of Elche have developed artificial intelligence solutions based on deep neural networks to predict traffic conditions using data from fixed sensors (such as loops) and connected vehicles. This new system makes it possible to predict traffic 15 minutes ahead of time.
To carry out this study, researchers of the UWICORE laboratory, which belongs to the I3E Centre for Engineering Research of the UMH, have digitised and implemented on the SUMO traffic simulation platform, a real traffic setting corresponding to a 97km stretch from Spain’s A-7 motorway, between the cities of Alicante and Murcia. They have worked with the collaboration of the Levante Traffic Management Centre, which provided data on all of its traffic sensors from the chosen stretch over a 12-year period.
This stretch has been chosen for its high influx of traffic (daily average intensity of 100,000 cars in places) and its high number of traffic sensors (99 in total), which make it possible to accurately measure traffic every minute. With a selection of this data, researchers have developed a digital simulation setting which makes it possible to very accurately generate the traffic endured by the A-7 stretch for 10 days. To do so, the UMH researchers have developed a new calibration methodology which enables the accurate and realistic generation of digital traffic simulation settings based on real data.
Until at least 15 per cent of vehicles are autonomous, there won’t be a noticeable benefit regarding the fluidity of traffic and the capabilities of motorways…”
With the digital traffic platform created at the UMH, researchers have developed techniques based on deep neural networks to predict traffic conditions 15 minutes ahead of time, using data from connected vehicles. Researchers have analysed how the insertion of the connected vehicle affects the accuracy of the traffic intensity, density and speed predictions. Their investigations have allowed them to prove that traffic prediction can be improved with data from just four per cent of the vehicles, compared to when the prediction is done with data from the traffic sensors that are currently deployed in the relevant A-7 stretch. The UMH researchers have also shown that the merging of data provided by the current traffic sensors with data from connected vehicles allows for an improvement in traffic prediction accuracy. For example, the merger of traffic sensor data from just 10 per cent of the vehicles decreases prediction error by 40 per cent compared to the traffic condition prediction done with data provided by the loops.
Head of the UWICORE group, Javier Gozálvez, explained: “Connected vehicles improve comfort and security, and boosts the digitisation of mobility. Furthermore, it also offers the public administrations and traffic managers new tools to know and manage the traffic. For example, with the data from connected vehicles, it is possible to learn the state of traffic and even predict it, without having to deploy and maintain traffic sensors as is done today. However, access to this data will have a cost, which means it is important for administrations and managers to know how many pieces of data they need to conduct their functions. The research of the UMH not only offers tools based on artificial intelligence for the characterisation and prediction of traffic conditions, but also make it possible to quantify the data necessary to be able to accurately predict traffic conditions. For example, the percentage of vehicles from which data is needed.”
The UMH research has been conducted in the framework of the PREDICT project (Prediction and characterisation of traffic with data from connected vehicles and autonomous vehicles), funded by the General Traffic Directorate. UMH researchers have also quantified the impact of autonomous vehicles on traffic as part of the project. Their conclusions show that until at least 15 per cent of vehicles are autonomous, there won’t be a noticeable benefit regarding the fluidity of traffic and the capabilities of motorways, unless solutions are developed to guarantee an efficient coexistence between autonomous and conventional vehicles.
Related topics
Artificial Intelligence, Traffic Management
Related cities
Spain
Related organisations
Miguel Hernández University (UMH)
Related people
Javier Gozálvez