How AI and machine learning enhances the safety, efficiency and passenger comfort of public transport
- Like
- Digg
- Del
- Tumblr
- VKontakte
- Buffer
- Love This
- Odnoklassniki
- Meneame
- Blogger
- Amazon
- Yahoo Mail
- Gmail
- AOL
- Newsvine
- HackerNews
- Evernote
- MySpace
- Mail.ru
- Viadeo
- Line
- Comments
- Yummly
- SMS
- Viber
- Telegram
- Subscribe
- Skype
- Facebook Messenger
- Kakao
- LiveJournal
- Yammer
- Edgar
- Fintel
- Mix
- Instapaper
- Copy Link
Posted: 17 October 2023 | Jan Haegeman - Nomad Digital, John Wright - Nomad Digital | No comments yet
Nomad Digital’s Head of Product Management, John Wright, and Product Manager, Jan Haegeman, share how the company is continuously innovating and collaborating with industry, utilising the latest artificial intelligence and machine learning technology to improve not only operational efficiencies within public transport, but also the passenger experience of travellers.
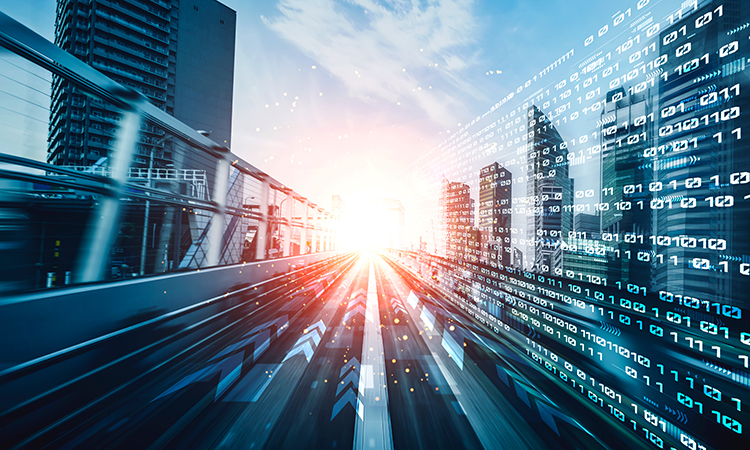
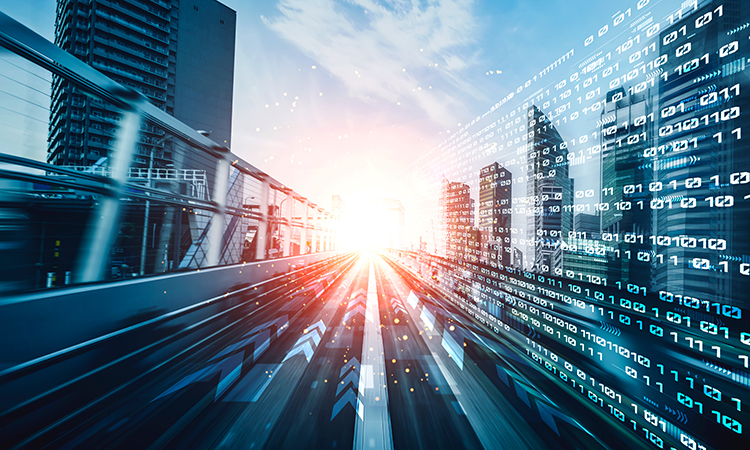
How has artificial intelligence and machine learning been integrated into rail and public transport systems to improve operational efficiency and contribute to maintenance and asset management?
Video analytics is bringing tangible benefits in these areas. Through collaboration, Nomad Digital is participating in work to monitor rail infrastructure using externally facing on-board cameras and monitoring systems in conjunction with enhanced GPS analytics provided by Cordel. This is being used to monitor the state of overhead catenary lines using Incremental Solutions OLErt system, pantograph equipment and trackside railway equipment, or to detect external situations like overhanging vegetation or graffiti on infrastructure.
By continuously monitoring the health of assets in real time, operators can anticipate issues, repair equipment before failures occur and avoid the resultant disruption to operations. We can also significantly reduce the need for ‘boots on ballast’, increasing the safety of track workers and reducing inspection time spent on-site outside of operational hours.
Nomad Digital is continuously working with several organisations who have developed video analytic solutions using existing and bespoke camera installations to support operators with asset management. The video data produced is transmitted across Nomad’s train to shore link to the cloud, where artificial intelligence (AI)/ machine learning (ML) technologies are harnessed to measure, detect defects or mark-up images and generate actionable reports.
Another example is in the area of passenger counting. We are working with an operator to train models to estimate passenger numbers based on passive scanning of devices connected to wireless radio access points, calibrated via a second source such as train weight, door sensors or on-board CCTV cameras. By correlating historical passenger numbers with weather data or information on holiday seasons or special events, we can measure the impact of such external influences on ridership and adapt services accordingly.
More extensive insights into ridership can be obtained when data can be related to individual, albeit anonymous, passengers. When we can infer movement around the train and embarkment or disembarkation, the operator can quantify, for example, use of a catering car, or develop an origin/ destination matrix showing passenger numbers for journeys between two specific stations. It can also help to inform passengers and station staff of the best location to stand on the station platform to avoid congestion and dispatch delays.
Finally, AI can be used to measure the knock-on effects of timetable disruptions and help to repair service by reinstating a regular interval to waiting passengers.
What about the passenger experience?
Our main focus is to improve the comfort and safety of the passenger throughout the journey.
As a multitude of sensors make a detailed real-time model of the passenger environment available, including temperature, humidity and air quality, operators can proactively fix issues before they impact the passenger experience.
Data on passenger numbers and carriage loading can be surfaced to passengers either via external signage on the train, platform infrastructure or even delivered directly to the passenger’s smartphone. Being informed in such a way means that the passenger can board directly in a carriage where space is available or move throughout the train to a carriage where a more comfortable experience can be found. Use of technologies like ChatGPT will increasingly accompany the passenger throughout their journey to provide tailored assistance to their needs.
When users provide feedback to the operator on any part of their passenger experience, from cleanliness of toilets or the friendliness of staff to train punctuality or the audibility of announcements, AI techniques can be used to perform sentiment analysis and benchmark operational performance.
Video analytics can detect and track luggage located on-board trains, helping to identify unattended or lost items. Passengers can be informed in real time of available storage space, either through announcements or notifications pushed to their smartphones, and can be warned when about to disembark without their luggage. Addressing the issue of abandoned or lost luggage can not only improve passenger safety and customer trust, but also reduce the operational impact of delay.
Similarly, optimising the distribution of luggage throughout the train can reduce dwell times by facilitating passenger exchange in stations. Using such analytics to track vacant carriage space for wheelchairs and mobility scooters can also improve accessibility and make the network available to all.
Can you provide examples of how AI and machine learning have had a significant impact on industry safety, security and reliability?
As mentioned in relation to asset management, operational reliability can be improved through predictive maintenance of train borne and trackside systems, analysing data to avoid in-service equipment failures and reduce downtime with Nomad collaborating with Incremental Solutions, Cordel and One Big Circle.
This can be based either on data collected via on-board sensors together with trained models able to estimate the time to failure, or else through video analytics, using cameras placed on entry to depots to identify, for example, deterioration of critical rolling stock components.
Passenger security can also benefit from advanced video analytics. Together with Dynamic Crowd Measurement (DCM), we’ve undertaken trials with a customer to use existing CCTV infrastructure to perform video analysis to monitor crowd flows in a station, measure densities on platforms and perform sentiment analysis through facial expression and behavioural recognition techniques.
This can allow an operator to proactively identify situations where passengers are showing signs of distress, either due to overcrowding or the development of a situation requiring intervention from staff to increase customer security or their feeling of well-being. The tracking which is performed doesn’t rely upon identification of individuals to address any data protection concerns and can provide operators with real time information to anticipate incidents and take preventive measures. These include filtering access to overcrowded areas or increasing service regularity to absorb increases in demand.
How do you see the roles of artificial intelligence and machine learning evolving in the future, and what innovations or developments can passengers expect to see in the coming years?
Whilst not all advances will be immediately visible to the passenger, there are several areas in which the passenger experience of travelling will be enhanced as AI techniques are employed to accompany the passenger from door-to-door.
This includes aid for navigating within stations and for planning multi-modal trips, with information being provided in advance for connecting journeys, whether by rail, bus, taxi or scooter. Integration of data from multiple systems will allow increasingly informed passengers to take a more holistic view of their travel requirements and plan their journey more comprehensively and more efficiently.
As greater numbers of on-board sensors and devices become network enabled, increasing amounts of data become available for aggregation, analysis and communication to both vehicle and remote systems. Many of these sensors provide information about the mechanical and electrical systems on the vehicle – e.g. brake wear, engine temperature or fluid levels – but, as noted above, an increasing number also monitor the passenger environment, including passenger counting devices, environmental sensors, seat occupancy sensors etc.
Using data from many sources provides the information for AI and ML algorithms to create alerts and predictions to dynamically make changes which improve both operations and the passenger experience.
One future development will be in the use made of such data, whether from sensors or CCTV images. Rather than simply recording data for subsequent review or post-mortem analysis, advanced algorithms will allow the characterisation and tracking of events which trigger particular situations; for example, equipment failure or passenger overcrowding.
In the field of video analytics, AI will be increasingly embedded into camera equipment to perform processing during recording.
With so much data available and powerful processing capability to hand, operators will be increasingly able to tailor this information to the specific circumstances of an individual passenger to give them a personalised experience addressing their particular needs.
To find out more or to speak to a member of the Nomad Digital team, contact them at [email protected].


John Wright is the Head of Product Management at Nomad Digital.
Jan Haegeman is Product Manager at Nomad Digital.
Related topics
Artificial Intelligence, Fleet Management & Maintenance, Passenger Experience, Public Transport, Security & Crime, Vehicle & Passenger Safety
Related modes
Rail
Related organisations
Cordel, Dynamic Crowd Measurement (DCM), Incremental Solutions, Nomad Digital, One Big Circle
Related people
Jan Haegeman, John Wright